Dayton, OH – In the ever-evolving landscape of artificial intelligence, certain individuals stand out as pioneers, pushing the boundaries of what's possible in this field. One such visionary is Dr. David Ménager, an AI research scientist at Parallax Advanced Research. Dr. Ménager's journey into the realm of cognitive AI systems began in Topeka, Kansas, where his childhood fascination with robots and AI ignited a lifelong passion. His educational background, which includes laboratory research at the University of Kansas under the mentorship of Dr. Dongkyu Choi and a Ph.D. in Computer Science, has molded him into the AI expert he is today. His work not only encompasses cutting-edge research but also a unique perspective on AI, one that emphasizes high-level cognition, structured knowledge representations, and a systems-level approach – all deeply rooted in an understanding of human cognition. As we delve into Dr. Ménager's career, we will explore his educational journey, his expertise in cognitive AI systems, and how his values align with Parallax's commitment to innovation and excellence.
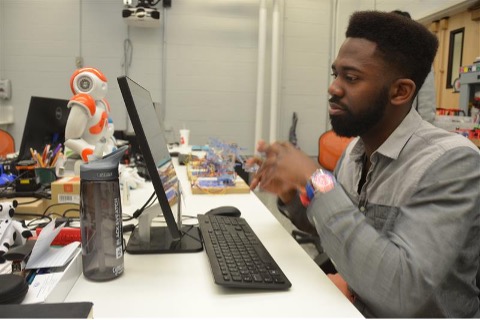
Q: Were there specific mentors or influential figures who played a significant role in shaping your research interests and career path?
Dr. Ménager: My journey in AI research has been greatly influenced by some remarkable individuals. During my time in graduate school, I had the privilege of being co-advised by Dr. Dongkyu Choi and Dr. Arvin Agah at the University of Kansas. Their mentorship and guidance were instrumental in shaping my research trajectory. Additionally, I've had the privilege of collaborating with David Aha at the Naval Research Laboratory, whose insights and expertise have been invaluable to my work. Beyond that, the incredible colleagues I have at Parallax Advanced Research continue to inspire and motivate me with their brilliance and camaraderie.
Q: Can you share any memorable moments or breakthroughs in your career that significantly impacted your research direction or approach?
Dr. Ménager: In the early stages of my work, I was deeply influenced by computational models of episodic memory, particularly those integrated within the Soar cognitive architecture, as well as incremental concept learning. During this period, I observed a significant gap in AI research – the understudied domain of event memory, with few computational models addressing it. As I delved into literature encompassing both psychological and computational perspectives on episodic memory, I came to appreciate its overarching importance. It became evident that numerous cognitive functions hinged on the retention of experiential content. What seemed to be missing in existing computational models of episodic memory was a comprehensive theory explaining how episodic knowledge transforms into long-term semantic structures. To address this, I drew inspiration from incremental concept formation systems like COBWEB, which construct probabilistic concept hierarchies through online learning from observed examples. This realization marked a significant turning point in my research approach and direction.
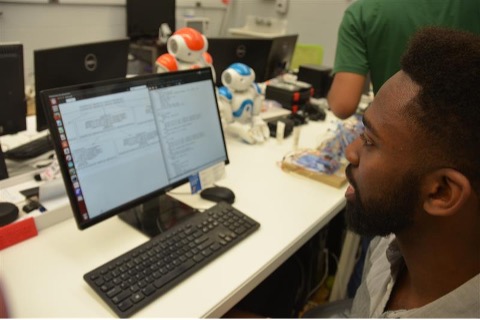
Q: What key lessons or insights have you gained throughout your career that you believe would benefit other researchers or professionals in the field?
Dr. Ménager: A valuable lesson I've learned, especially applicable to junior researchers and professionals in non-academic roles like mine, is the importance of breaking down your research agenda into manageable chunks. When opportunities arise, seize them to work on specific aspects of your research agenda incrementally. By doing so, you can steadily advance towards your overarching goals, one piece at a time. This approach allows for meaningful progress even within constraints and provides a path to realizing your research ambitions.
Q: What are your long-term goals and aspirations in your field of expertise, and how do you plan to contribute to the advancement of Cognitive AI Systems in the coming years?
Dr. Ménager: When I think about my long-term goals and aspirations, two areas of particular interest stand out. First and foremost, I am deeply invested in the development of event memory-enabled AI systems for long-duration autonomy. In environments where sustained autonomy is essential, such as space exploration missions or unmanned underwater vehicles, AI systems must operate autonomously for extended periods with minimal human intervention. To achieve this, these AI systems should possess the ability to learn and adapt their knowledge representations based on their interactions with the environment. Furthermore, they should be proficient in reporting relevant information to humans, ensuring transparency and trust in their decision-making processes. My research in event memory is well-suited to contribute to both the learning and explanation aspects of these autonomous systems.
Additionally, I have a vision for advancing multi-agent systems, such as teams of drones, through the implementation of shared event memory. By integrating a common event memory system across a team of agents, we can substantially enhance their collective performance in diverse scenarios and environments. This approach enables agents to exchange information and leverage one another's experiential knowledge. A particularly fascinating challenge lies in adapting this concept to heterogeneous platforms with varying sensing capabilities, thereby enhancing the synergy between different agents in the system. These are the avenues through which I aim to contribute to the continued advancement of Cognitive AI Systems in the years to come.
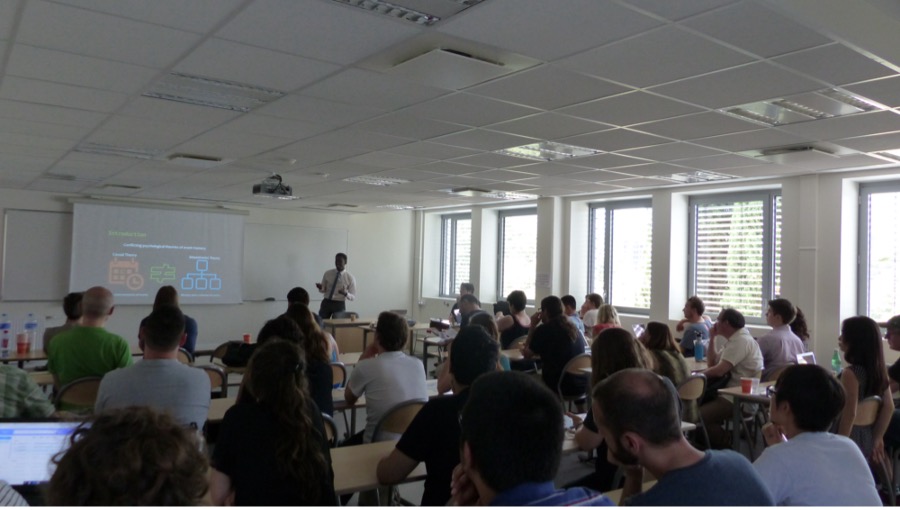
Q: What initiatives or projects are you currently involved in at Parallax?
Dr. Ménager: My current focus at Parallax revolves around contributing to two R&D projects: DARPA SCEPTER and DARPA In the Moment. The SCEPTER program is directed towards the development of an AI tool tailored for human military planners. This tool's primary function is to generate machine-generated strategies for accomplishing objectives within a combat environment. The aim here is to empower military decision-makers with advanced AI-driven strategic planning capabilities.
On the other hand, the DARPA In the Moment project is centered around equipping AI systems with the ability to model the decision-making processes of trusted human experts, particularly in the context of combat triage and mass casualty situations. This initiative seeks to enhance the efficiency and effectiveness of decision-making in critical and high-pressure scenarios.
Both projects are at the forefront of cutting-edge AI research and have the potential to make significant contributions to our understanding and application of AI in real-world, high-stakes situations.
Q: What do you believe are the key future directions or advancements that can be expected in the field of Cognitive AI Systems?
Dr. Ménager: I foresee a pivotal shift towards harnessing the potential of data-driven approaches within the framework of higher-level symbolic processes. A notable example of this evolution is the exploration of how large language models can be effectively controlled and integrated into cognitive architectures. Additionally, there is significant promise in the development of methods for learning structured representations from unstructured data sources, including text, images, and sensor data. These advancements hold the potential to revolutionize the capabilities of cognitive AI systems, enabling them to excel in complex tasks that require a synthesis of symbolic reasoning and data-driven insights.
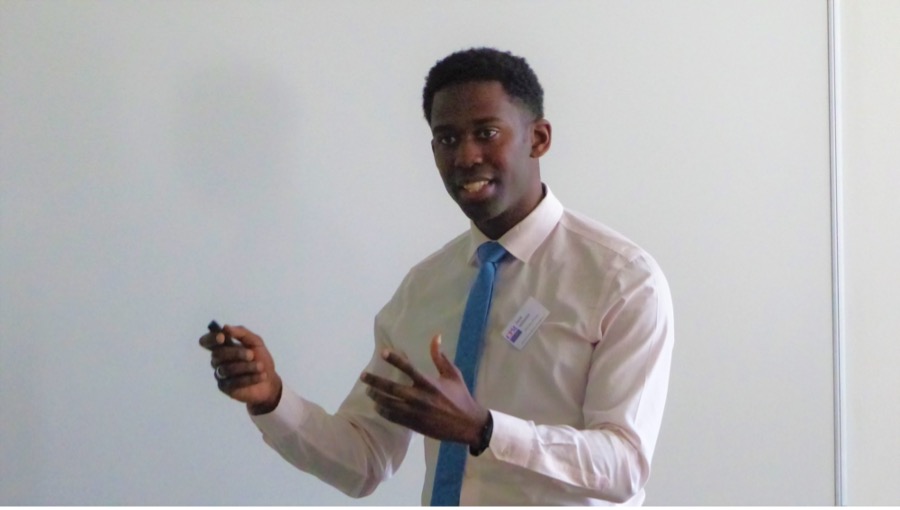
Q: You recently published a research article in Case-Based Reasoning Research and Development. Can you provide an overview of your published research, its significance in the field, and the specific real-world problems or scenarios it aims to solve?
Dr. Ménager: This research addresses a vital aspect of AI: estimating the state of structured domains based on available measurements, a fundamental challenge. It employs an integrated event memory system within a cognitive architecture, enabling agents to learn and represent extensive state probability distributions. The novel use of rule-based representations facilitates near real-time execution. Its significance lies in practicality, especially in scenarios with partial information. It offers a robust solution for real-world state estimation challenges, showcased through experiments in the Minecraft domain. This work advances AI by providing a novel approach with broad applications in intelligent state estimation.
Q: What makes your approach or techniques in your publication novel and different from existing methods?
Dr. Ménager: Our approach stands out due to its ability to tackle complex state estimation problems involving large joint probability distributions. Unlike traditional methods that face challenges with the exponential growth of possible outcomes, we introduce a Bayesian network representation that imparts structure to these distributions. This structural simplification allows us to handle estimations involving tens or hundreds of variables with greater ease. Moreover, our system learns the Bayesian network structure as it observes examples, resulting in improved predictive performance over time. This combination of scalability and adaptive learning sets our approach apart from existing methods.
Q: What motivated you to choose the theme/topic of your research?
Dr. Ménager: My research theme aligns with the broader field of event memory research. It's driven by the idea of demonstrating how our event memory system can effectively store, retrieve, and make predictions based on past experiences. This foundational work paves the way for supporting various higher-level cognitive tasks that heavily depend on this capability.
Q: Could you share any interesting challenges or obstacles you faced while conducting this research, and how did you overcome them?
Dr. Ménager: During the experimentation phase of this research, I encountered a rather unexpected challenge. It turned out that my event memory system, which was designed to efficiently handle data, was overwhelming the hardware memory resources of my computer. Despite the Bayesian network representation being a compact way to encode joint distributions, the sheer scale and complexity of the Minecraft game posed difficulties in capturing all the dynamics.
To address this, I explored an innovative solution by incorporating rule-based representations. Conventionally, Bayesian networks use multi-dimensional tables to represent conditional distributions, which can be problematic when dealing with sparse data or higher dimensions. By encoding slices of these tables as rules, I was able to capture the local structure and significantly reduce the data's footprint. This transformation ultimately enabled the system to scale effectively within the Minecraft domain, allowing me to successfully complete the experiments.
Q: Has your research led to any unexpected or exciting discoveries or applications beyond the initial scope of your publication?
Dr. Ménager: My research has given rise to a particularly exciting application within my involvement in DARPA's In the Moment Program. During the proposal phase of this program, Parallax put forth a system with the capability to assess the diagnostic value of specific decisions. In response to this challenge, I've been able to leverage my event memory system to perform state estimation. This extends its functionality to infer the mechanisms of injury for casualties and provide valuable metrics regarding which decisions yield the most significant information gain about a casualty's true condition. This unanticipated application demonstrates the versatility and real-world impact of the research beyond its initial scope.
Are you interested in a STEM career with a dynamic nonprofit research institute? Join our team. View our job vacancies here.
###
About Parallax Advanced Research
Parallax Advanced Research is a 501(c)(3) private nonprofit research institute that tackles global challenges through strategic partnerships with government, industry, and academia. It accelerates innovation, addresses critical global issues, and develops groundbreaking ideas with its partners. With offices in Ohio and Virginia, Parallax aims to deliver new solutions and speed them to market. In 2023, Parallax and the Ohio Aerospace Institute formed a collaborative affiliation to drive innovation and technological advancements in Ohio and for the Nation. Ohio Aerospace Institute plays a pivotal role in advancing the aerospace industry in Ohio and the nation by fostering collaborations between universities, aerospace industries, and government organizations and managing aerospace research, education, and workforce development projects. More information about both organizations can be found at the Parallax and OAI websites.